Harness Data to Unlock Innovation and New Revenue Opportunities
HCLTech’s next-generation Data Engineering and AI services enable enterprises to accelerate digital transformation, unleashing the power of real-time data and advanced analytics to innovate data-centric products, embrace industry 4.0 and effectively leverage disruptive technologies.
A trusted ally for digital transformation initiatives, HCLTech provides a robust data foundation of scalable, speedy and reliable systems that ensure the products, services and operations they support deliver maximum value to every user.

Accelerating Data Modernization and Data-Centric Product Development
Our proven solutions and frameworks are designed to simplify enterprise data modernization and fast-track value creation. This accelerates time-to-market for next-generation data-centric products and services.
HCLTech Graviton
Data platform modernization framework that enables enterprises to efficiently build, deploy, monitor and maintain agile and cost-efficient, modern data platforms.
HCLTech AION
AI lifecycle management platform for applying machine learning to real-world problems. AION automates the complete data pipeline from raw dataset ingestion to deployable machine learning model with a low-code/no-code approach.
HCLTech Cognitive Knowledge Assistant (CKA)
A one-stop solution for enterprises embracing GenAI. Pre-bundled with use cases such as chatbot, code generation and summarization. Highly scalable system that features a built-in knowledge graph and integrates with both open-source and SaaS LLMs.
HCLTech Antares iDL
Modern data platform with integrated components for building scalable industrial data platforms using best-of-breed technologies, designed for the cloud era.
HCLTech Data Genie
Synthetic data generation for structured and unstructured data. Works for a variety of data types including images, video, time-series and tabular.
HCLTech Spotter DQV
Data quality monitoring solution for the detection and correction of data quality issues. Uses a standard framework-driven approach for efficiency and leverages advanced ML/DL models for outlier detection, data imputation and variance anomaly.
HCLTech Elements for Tableau
Enterprise-grade write-back and data annotation. Enables users to comment on and overwrite data directly inside dashboards, accelerating communication around data and improving decision speed and quality.
Service Offerings
AI and ML services
- ML and NLP services
- MLOps
- Computer vision
- Deep learning
- Data visualization and augmented analytics
- Generative AI services
Data engineering consulting
- Data modernization roadmap
- Data engineering platform selection
- Analytics enablement
- AI strategy development
- AI/ML operationalization
Data modernization and migration
- Cloud data engineering services
- Modern data pipelines
- Cloud data migration services
- Data lakes
- Cloud data platforms
Data marketplace and governance
- Data exchanges and marketplaces
- Data quality and stewardship
- Data literacy
- Data cataloging
- Data mesh
DataOps
- Data platform maintenance
- Data observability platform
- Data pipelines support
- Data application support
Case Studies
Trends and Insights
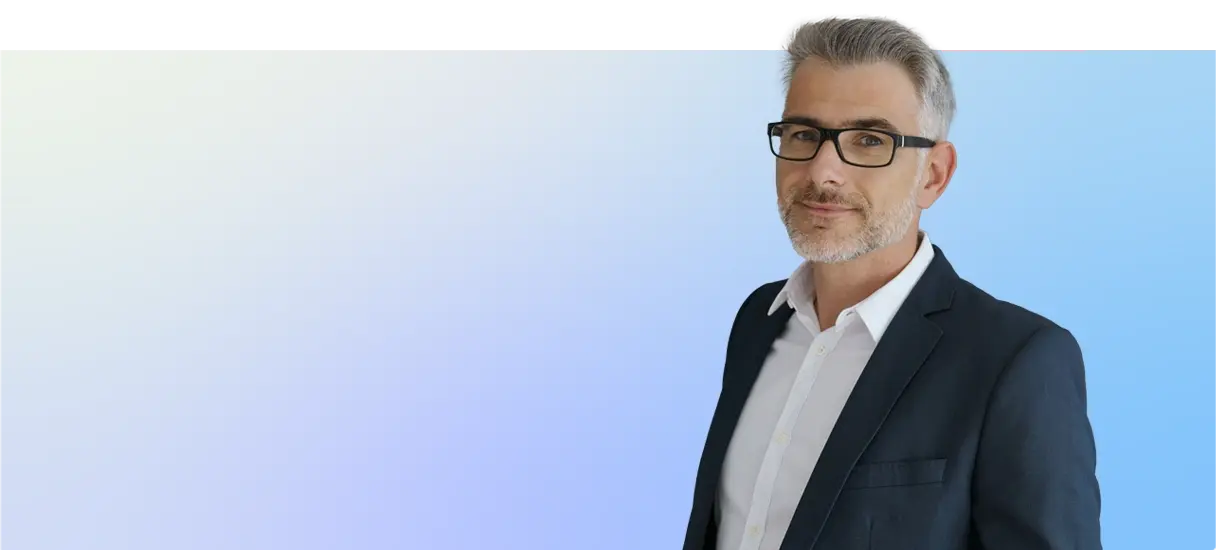
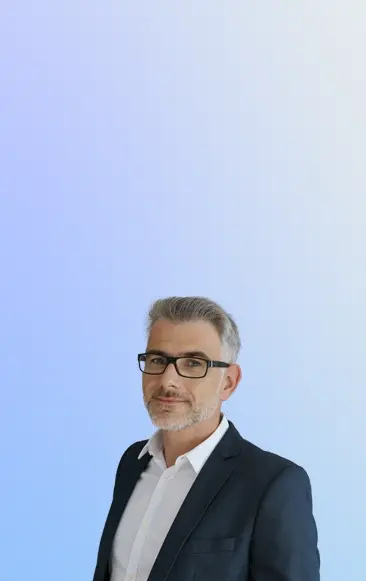
Talk to our experts
Accelerate your data modernization journey with HCLTech’s comprehensive suite of data solutions for value creation.